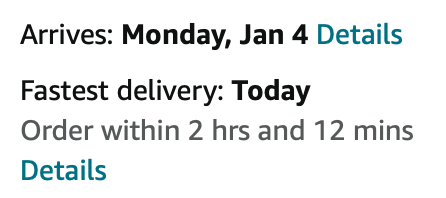
Connecting Pre-Purchase Promise to Shipping Selection
Who is this for?
Read this if you are an operations leader looking for the best way to marry upfront delivery promises before purchase with the processes of fulfillment and shipping after the purchase.
Summary
You know the phrase about the chicken or the egg. In an ecommerce supply chain world, the prompt fits when debating where to pick shipping methods and how to promise the results to customers. The right answer is surprising and provocative, blending what you would expect with curveballs you do not. This play will walk you through the operational side of keeping pre- and post-purchase promises to customers.
Overview
A good shopping experience requires promises made by retailers to consumers.
Retailers are, surprisingly, slowly getting better at communicating this kind of estimate on product description pages to customers.
The best retailers in the world position it as a promise, or, as the team at Saks OFF 5TH recently launched in November 2022, a “delivery guarantee”. Call it what you will, but the goal is to set customer expectations upfront before a purchase, which has proven to boost cart conversion rates.
As well as improve customer loyalty and word of mouth advertising, both critical to profitability in today's industry.
But how do you accurately project a delivery promise before purchase based on dynamic fulfillment constraints that are only understood after an order is placed? That's the question at heart in this play.
Common convention compels operators to work backwards from the shipping constraint. The typical thinking with a simplified example goes "If we only have a 5-day ground method available, then we can't promise delivery faster than 5 days." In effect, those who start there are locking themselves into upstream decisions based on the assumption that downstream constraints are static.
Those who start here aren't wrong, but they are only directionally right for a simple reason. The upper bound might be hard capped by policy (very rare is a delivery later than its method's SLA), but the lower bound can be dynamic (a 3-day method might still deliver the next day based on geography).
In truth, it's a bad idea to break this important supply chain principle: Never make decisions before they need to be made. Doing so, like selecting the downstream shipping method all the way up at the product description page, creates unexpected constraints and actually increases the likelihood a promise won't be met resulting in a poor customer experience.
Instead, set an upfront promise based on a probabilistic guess, then keep your operational processes flexible enough to adjust so that the promise isn't broken.
The rest of this play will explain how to do just that.
Flat Pricing is Best
Another common convention is to pass through shipping prices to customers. The thinking goes that if shipping for a unique order costs $13.58, it's better to ensure shipping is priced accordingly upfront as $13.58 so as to not lose any margin.
It's a reasonable thought. In fact, this was an angle at Amazon for a while in the early days until enough data and hypothesis testing showed the opposite was better along key metrics such as conversion rates, cost-per-unit, margin, and customer loyalty. It turns out a flat rate pricing scheme for shipping is preferred by consumers, while also making backend operations easier—an unexpected win-win. It shocked us then, but has now become a more common industry-wide convention today.
It's much better to create shipping tiers based on policies, then attach base flat rate prices to those tiers, which then all customers receive as options. There is certainly room for variation and customizations, such as cart promotions ("Free shipping on orders over $79") or upgraded prices based on geography (a customer in the same state as the FC might be upgraded to the fastest tier for free because it's actually the same slower method, and thus same cost, behind the scenes). But the idea is to spread a base flat rate per tier across all customers and SKUs.
There is no firm rule on how to structure your tiers or prices. In fact, it's best to do an analysis of your business requirements and customer profile to set your policies. For example, maybe a high-margin luxury jewelry retailer will always provide free 2-day shipping because it's a highly competitive space and the business can eat the cost to ship a small light package priced in the $1000s. Or, on the flip side, an appliance outlet might have three tiers—Next-Day, 3-Day, 5-Day—with dynamic prices passed through to customers due to the high costs of shipping big and bulky items. And anything in between those two polar opposites.
In general, with exceptions only on the fringes, everyone should strive for flat rates, with the specifics of your business setting the prices. If your shipping profile consists of bigger dimweights and/or longer delivery distances, you might price the 5-Day option around $10 whereas if dimweights are consistently smaller and you have inventory placed more closely to potential customers, it might be $6. (More on shipping profiles and carrier rates here.)
Let's pretend these are the prices for our three tiers offered to customers for the remainder of the article.
A key insight is to not map each option to a specific shipping method. For example, this should not be the operational policy on the backend with logic setup to support it:
Instead, consider the three options as the promise made to the customer upfront. You are promising to the customer to deliver it "fast", "kinda fast", "not that fast but super cheap" to which the customer will pick one of the three experiences.
This goes hand-in-hand with the How to Design an End-to-End Delivery Promise play. With these options in place, you do not communicate to customers a method or shipper in the interface. Instead—and this is where it gets hard—you provide estimated delivery dates for each of the three options.
A note on free shipping
This is a topic for a different, longer play in the playbook, but consumers hate paying for shipping.
While at Amazon, there were periods where we saw over 95% of customers opted for free shipping even when it took longer to deliver, which mirrored what colleagues at other big ecommerce stores said they saw, too.
Don't just trust our anecdotes that consumers hate to pay for shipping. The yearly drip of industry reports prove the point too. The strongest recent evidence comes from the National Retail Foundation (NRF) 2019 Consumer Report:
- 60% of consumers expect free standard shipping and will shop elsewhere without it.
- 75% of consumers expect free shipping on orders over $50.
- 68% of consumers look up shipping costs before placing an order.
- 39% of consumers expect free 2-day shipping.
- 29% of consumers back out of a purchase because the retailer did not offer free 2-day shipping.
In the future, you as an ecommerce operator will want to pair this play with a "How to Provide a Free Shipping Program" (which has yet to be published) because one of the shipping options offered should be a free tier.
Probabilistic guesses are how to do the estimate
Now that we've established the idea that each shipping option is an abstracted concept which carries through the rest of the fulfillment process, the next piece to understand is how an estimated delivery date for the method is created.
The answer is data science.
Using historical data, it's straight forward to construct a model that uses several dynamic inputs, such as:
- SKU
- Dimweights
- Box options
- FC locations
- Carrier contracts
- Customer destination
The model makes it easy to predict an estimated delivery date at the order level.
Geography matters the most. Let's look at an example.
Let's say you have one FC on the West Coast, and a customer on the East Coast is checking out. We know from historical data that the "fast" option, which aims for a 2-Day delivery, will need to be an expensive overnight air method selection, while the other options will correlate with basic methods.
In this scenario, you will want to show your "base" shipping options and prices because there are no real opportunities for upgraded arbitrage.
Meanwhile, let's say a different customer in California is checking out with the same SKU located at the West Coast FC in her cart.
In this case, you know that the slowest and cheapest option will actually be delivered much more quickly. Consequently, you can update the shipping options to charge the "base" price for the slowest shipping, but change the interface so the customer can select free upgraded shipping to the fastest tier.
The key insight is that you are not assuming the fastest promise is tied to the most expensive and fastest method guarantee. Instead, you are making a fast promise, but because of historical data modeling, you know that the promise can be met with the cheapest possible shipping method.
Importance of knowing the customer (ZIP)
Since presenting a delivery promise to a customer on a product description page is based on a model, the calculation is dependent upon a few key inputs. The most important input that companies don't have control over is customer information, specifically the delivery destination location.
You can solve this a couple different ways.
The most dependable is by ensuring the customer is logged into an account on the store because a presumed destination address can be managed there. The address might be a default address added during account creation, or an address used in a prior order. Quick example from Amazon:
If the customer is not logged into an account, the next fall back is to prompt the customer to indicate a general geography. Omnichannel retailers often do that by asking the person to "pick their local store" via zip code.
As a last resort, you can make assumptions based on IP address.
PDP vs. Cart estimations
It's critical to make SKU-level estimations on a product description page (PDP) and cart-level estimations during checkout. Do not mix the two.
For example, if a customer already has two items in her cart, and is looking at a third item, do no make a delivery promise on the third item's PDP that is estimating for the full order. Keep it specific to the SKU being viewed.
Why? The answer is variability. If doing a cart-level estimation on a PDP, the customer might edit the cart after the fact, thus changing the estimation.
Instead, it's best to always show an estimation and a promise for a specific SKU on that SKU's PDP. Then, when the customer checks out, you show shopping options for the full order.
The cart-level "fulfillment plan"
The customer checks out. Now what?
Think of the fulfillment process as managing a "cart-level fulfillment plan" for that order. Here are the logical steps:
Select location(s)
Take stock of current inventory levels and calculate the best location to deliver the order. It's usually from a single location, but sometimes splitting the order makes sense. The most important consideration is location to customer, meaning short-haul shipments are cheaper and faster than long-haul shipments. Often, selecting the location consists of analyzing the other properties of the fulfillment plan, such as probable box sizes and methods, but also FC-specific properties such as cut-off times or inventory levels because all those factor into the criteria supporting the best decision for the problem "How do I ship this order at the cheapest possible price while hitting the promise we made prior to purchase?"
Guess the probable method
With a location selection, make a probable guess to the best shipping method from that location. Note you aren't actually picking that method and printing a label yet, but making a probable guess to inform the rest of the decisions being made.
Guess box sizes
With a method probability selected, decide which box size to use (or sizes of splits). This matters because it informs method rates. Some FCs might have different box sizes available which is why this is a dynamic real-time assessment.
Send the order to the FC(s) for pick-and-pack
With network-level logic completed upfront, send the order to the FC(s) for completion. Normal pick and pack processes are completed.
Now select the shipping method at the last possible moment
The reason to place this process step at the very end is to ensure flexibility to keep a promise.
Most of the time, the probabilistic guess made pre-purchase and then again during instantiation of the "fulfillment plan" will carry through to completion of this step. But sometimes, delays happen. Maybe it was related to weather, labor, inventory, accidents, just missing the cut-off time, or any other unexpected bottleneck. Regardless the reason, selecting the method and printing the label as the very last step before handing the package off to the carrier allows for the ability to upgrade shipping if needed in order to meet the promise made.
Rest assured, the small margin hit for upgraded shipping for these periodic delays is money well spent. Data proved time and again at Amazon that the benefits of continual customer loyalty outweigh the added cost. What matters to the customer is transparency and that you keep your promises.
Incidentally, this is a perfect example to illustrate the importance to have many carrier shipping options available at each FC location. The more options available, the greater the chance for cost-saving arbitrage plays, but also the greater the chance that you can find an upgraded method to meet a promise that won't be too much of a financial hit. We talk about adding and managing multiple carriers in this play.
Gotchas
Marketplaces + Merchants
If your business is a marketplace model with many merchants, the plays discussed above differ slightly.
Think of each merchant as an extra step which adds its own time to the projected promise. The key is to manage an up-to-date database of merchant performance and include the measurements in the data model. Maybe Merchant ABC is reliably fast and always packs and ships an order under 24 hours, whereas Merchant XYZ is unreliable. In a situation like this, adding an extra day to a promise for a SKU sold by Merchant ABC is a confident way to manage a promise. With Merchant XYZ, however, the inability to reliably fulfill an order quickly means the merchant is either penalized with a longer estimated delivery date, or not included at all in a projected promise.
The key is to manage the merchants in a dataset were performance on every order can be continuously updated.
What to do without data
Since the entirety of this approach hinges on sophisticated modeling using solid data producing confident estimations, not having good data or any data at all will break the play.
In this scenario, the answer is straightforward: Prioritize collecting the data first, then gradually architect in a delivery promise concept.
What matters is continual performance data on an order-by-order basis, including:
- SKU information
- FC process information
- Carrier information
- Merchant information (if a marketplace)
For the average ecommerce retailer, roughly 6 months of data is enough to produce a model that will produce the necessary results.
Policy-driven fulfillment
Ecommerce fulfillment is ultimately a collection of policies. For streamlining delivery promises, the policy that matters most is setting an upper bound.
Be sure to decide as a company the right upper bound. It could be 3 days, 5 days, 15 days—whatever. But it should be static, and it should drive all processes.
How Shipium Helps
There are three key moments discussed in this article where sophisticated data science and complex logic make the magic happen.
The first is the delivery promise data modeling on the PDP and cart checkout workflows. Shipium's platform manages this process for you and makes the job as simple as an API call.
The second is the "fulfillment plan" logic across a set of multiple nodes (FCs, Carriers, SKUs, Boxes). Shipium's platform performs that duty for you.
The third is selecting the best carrier method at then end of the fulfillment process. The real-time factors which go into that decision are complicated to manage. Shipium's platform makes that decision as simple as an API call as well.